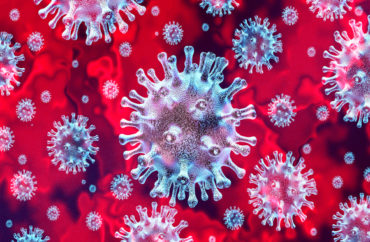
‘Epidemiological modelers haven’t adequately adapted their estimates’
You can run around preaching that the sky is falling, or you can look at the numbers we already have and make intelligent forecasts.
Joining their colleague John Ioannidis in throwing cold water on coronavirus conventional wisdom, professors at the Stanford University School of Medicine warn that the apocalyptic figures thrown around for COVID-19 in America “could plausibly be orders of magnitude too high.”
Eran Bendavid and Jay Bhattacharya write in The Wall Street Journal that we are focused on the wrong statistic: deaths from identified cases. Because of “selection bias in testing” and limited data on the extent of that bias, the possible fatality range is too great to be meaningful.
They find it “plausible but likely” from available figures that the more useful statistic – the infection rate – is “orders of magnitude larger” than thought, meaning deaths following infection are quite low, relatively speaking.
If testing of evacuees from Wuhan matches the infection rate of “greater Wuhan,” a population of 20 million, then the infection rate was “about 30-fold more” than reported cases, meaning a fatality rate “at least 10-fold lower” than estimates.
An Italian town of 3,300 that got fully tested showed a “prevalence rate” of 2.7 percent, which extrapolated to its province would mean 26,000 infections compared to 198 identified cases. Italy’s fatality rate could actually be closed to 0.06 percent, Bendavid and Bhattacharya say.
Based on the admittedly unrepresentative test sample of NBA players, where player contact could have made the infection rate higher, the “lower-bound assumptions” extended to cities with NBA teams would mean an infection rate 72-fold higher than identified cases across the U.S.
MORE: Stanford epidemiologist warns crackdown is based on bad data
Our panic stems from two misleading factors. Tests don’t catch people who recovered from infections, and they were “typically reserved for the severely ill” until recently:
Together, these facts imply that the confirmed cases are likely orders of magnitude less than the true number of infections. Epidemiological modelers haven’t adequately adapted their estimates to account for these factors. …
An epidemic seed on Jan. 1 [based on tens of thousands of travelers from Wuhan in December] implies that by March 9 about six million people in the U.S. would have been infected. As of March 23, according to the Centers for Disease Control and Prevention, there were 499 Covid-19 deaths in the U.S. If our surmise of six million cases is accurate, that’s a mortality rate of 0.01%, assuming a two week lag between infection and death. This is one-tenth of the flu mortality rate of 0.1%. Such a low death rate would be cause for optimism.
One-tenth the flu mortality rate.
We’ve been making extreme decisions based on incomplete and misleading data, as Ioannidis says, and his colleagues Bendavid and Bhattacharya emphasize the difference between an epidemic that kills 20,000-40,000 and two million.
Antibody testing needs to be ramped up to provide more useful data than the highly misleading rate based on identified (known sick) cases:
If we’re right about the limited scale of the epidemic, then measures focused on older populations and hospitals are sensible. Elective procedures will need to be rescheduled. Hospital resources will need to be reallocated to care for critically ill patients. Triage will need to improve. And policy makers will need to focus on reducing risks for older adults and people with underlying medical conditions.
We need to “evaluate the empirical basis” of the lockdown strategy embraced by the nation’s mayors and governors, because it could be imposing entirely avoidable costs on “the economy, community and individual mental and physical health.”
MORE: Public health professors warn the cure could be worse than the disease
IMAGE: Lightspring/Shutterstock
Like The College Fix on Facebook / Follow us on Twitter

Please join the conversation about our stories on Facebook, Twitter, Instagram, Reddit, MeWe, Rumble, Gab, Minds and Gettr.